Unlocking the Power of Data Labeling in Machine Learning
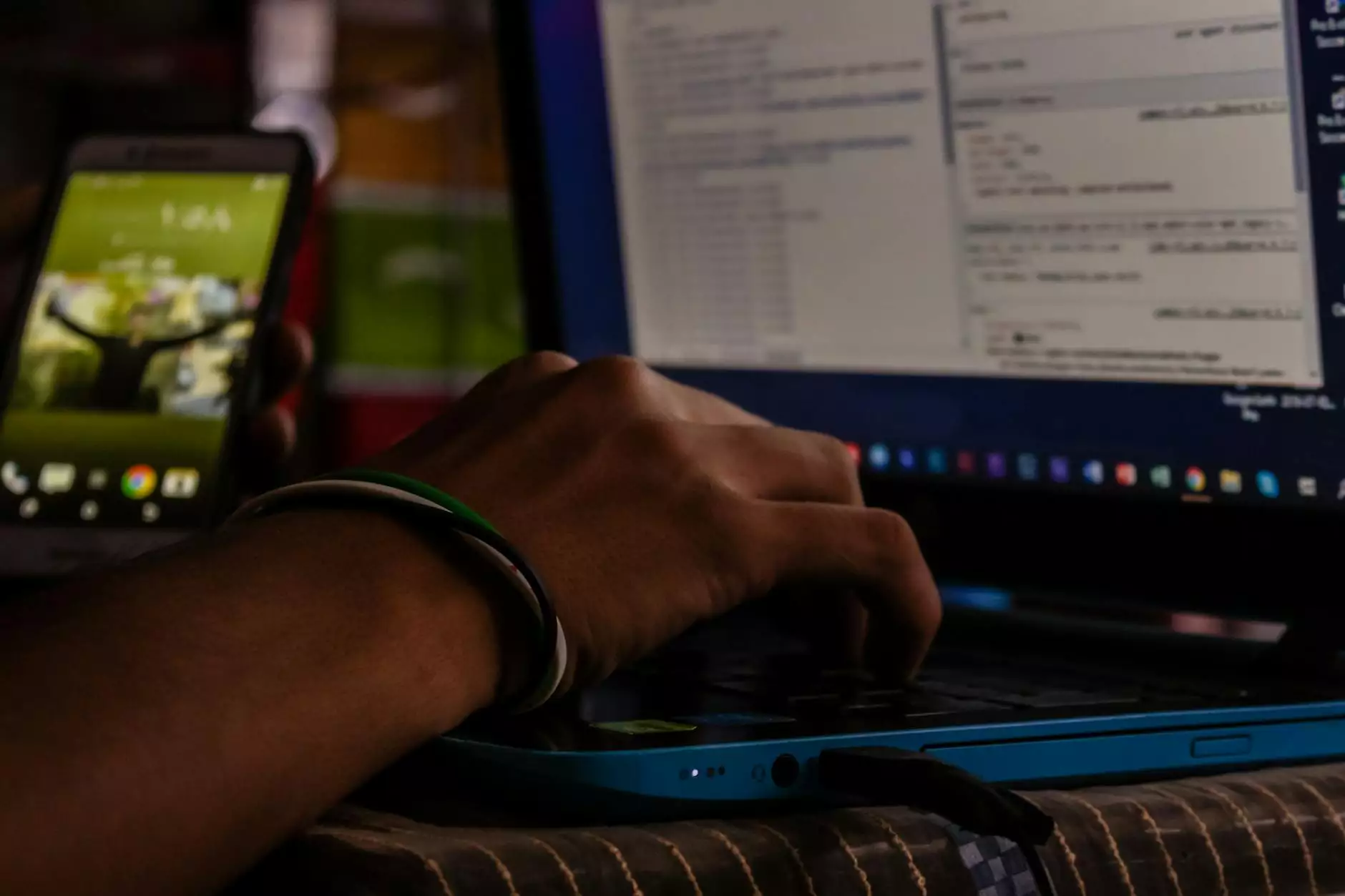
In today’s digital age, the significance of data labeling cannot be overstated. It serves as the backbone of machine learning, enabling algorithms to learn from data and make intelligent decisions. Without accurate labeling, machine learning models would be ineffective, limited in their ability to perform tasks such as image recognition, natural language processing, and more.
Understanding Data Labeling and Its Importance
Data labeling refers to the process of annotating or tagging data to make it understandable for machine learning algorithms. This encompasses a diverse set of practices depending on the type of data being handled, including:
- Image Annotation: Tagging objects in images for image recognition tasks.
- Text Annotation: Labeling text for sentiment analysis, named entity recognition, and other NLP applications.
- Audio Annotation: Identifying sounds, transcribing speech, or labeling audio segments for various applications.
- Video Annotation: Annotating video frames to enable object detection or activity recognition.
This process creates labeled datasets that serve as the training data for machine learning models. The quality of these datasets directly impacts the performance of the models built upon them. Quality data makes for effective machine learning, while poorly labeled data can lead to errors, misinterpretations, and ultimately flawed conclusions by the algorithm.
The Role of Data Annotation Tools
As businesses increasingly rely on machine learning, the demand for robust data annotation tools and platforms grows. These tools automate and streamline the data labeling process, allowing organizations to scale their machine learning initiatives effectively.
Key Features of a Powerful Data Annotation Tool
A comprehensive data annotation tool should offer several key features:
- User-Friendly Interface: Easy navigation is crucial for efficiency, allowing annotators to work quickly and accurately.
- Collaboration Capabilities: Teams often work in parallel, so a tool must support real-time collaboration to enhance productivity.
- Quality Control Mechanisms: Features that ensure the accuracy of labeled data, such as double-checking annotations or machine learning-assisted suggestions.
- Customizability: The ability to tailor the tool to specific project needs, including different labeling formats and tasks.
- Integration APIs: Seamless integration with existing machine learning frameworks and workflows to enhance data processing pipelines.
Why Businesses Should Prioritize Data Annotation
Investing in high-quality data labeling is crucial for various reasons:
1. Improved Model Accuracy
Correctly labeled data is foundational for creating accurate machine learning models. High-quality datasets lead to better predictions, which are essential for applications like fraud detection, customer segmentation, and predictive maintenance.
2. Enhanced Decision-Making
With reliable machine learning models, businesses can make data-driven decisions. For instance, accurate customer behavioral predictions can lead to improved marketing strategies and enhanced customer experiences.
3. Competitive Advantage
Organizations that effectively implement machine learning through proper data labeling can achieve a competitive edge in their respective markets. Whether in healthcare, finance, or retail, data-driven insights can lead to innovative solutions and exceptional customer service.
4. Cost Efficiency
Although the initial investment in data labeling may seem high, the long-term savings and increased profits from accurate models far outweigh the costs. Well-implemented ML models reduce operational errors and increase productivity, ultimately leading to cost savings.
Keylabs.ai: Leading the Charge in Data Annotation
At Keylabs.ai, we understand the criticality of data labeling in machine learning. Our data annotation platform is designed to meet the diverse needs of modern businesses. Here’s how we excel in providing data labeling services:
Scalability
Whether you're a startup or an established enterprise, our platform scales with your needs. We handle projects of any size, ensuring that your data labeling machine learning endeavors can expand without a hitch.
Expert Annotators
Our team of expert annotators is trained to ensure that data is labeled accurately and consistently. We believe that human oversight, coupled with machine support, yields the best outcomes.
Versatile Data Handling
From text and audio files to images and videos, we provide comprehensive services that meet all your data annotation needs. Keylabs.ai ensures that no matter the data type, labeling is performed with precision.
Advanced Quality Assurance
Our rigorous quality assurance processes guarantee that every labeled dataset meets the highest standards. We utilize both automated checks and expert review to maintain data integrity.
Challenges in Data Labeling
While data labeling is essential, it does come with its challenges:
1. Time Consumption
The data annotation process can be time-consuming, especially for extensive datasets. It requires meticulous attention to detail and considerable manpower, which can strain resources.
2. Complexity of Data
As data types become increasingly complex, the labeling process also evolves. For example, accurately annotating nuanced sentiments in text or identifying intricate patterns in images can be particularly challenging.
3. Maintaining Consistency
Consistency across annotations is crucial for high-quality datasets. Variability in annotation can lead to model performance issues, which businesses must carefully monitor and manage.
The Future of Data Annotation and Machine Learning
The future of data labeling in machine learning looks promising. As technology advances, we can expect to see improvements in automation and AI-assisted annotation processes, which will help address current challenges.
1. Increased Use of AI in Annotation
Machine learning models can assist in the labeling process, identifying patterns and suggesting annotations that human annotators can refine, effectively accelerating the process.
2. Enhanced Collaboration Tools
The tools that support data annotation will continue to improve, offering better collaboration features, real-time tracking, and integration with machine learning pipelines.
3. Focus on Ethical Data Usage
As data privacy regulations become more stringent, ethical data labeling practices will gain importance. Businesses must prioritize transparency and ethical considerations in their data labeling processes.
Conclusion
In conclusion, the importance of data labeling in machine learning cannot be overstated. It serves as the foundation for developing accurate, reliable models that drive decision-making in today’s data-driven world. By leveraging advanced data annotation tools like those provided by Keylabs.ai, businesses can enhance their efficiency, improve model accuracy, and unlock valuable insights that propel them ahead of the competition.
In an era where data is the new oil, proper data labeling is the refining process that enables businesses to extract meaningful, actionable intelligence from raw data.